Authors: Vivek Sharma and Dr. Sumita Kumari
Plant breeding programmes used to the large number of germplasms to identify lots of quantitative variation to deeply analyzed. Statistical methods applied for design, experimental tests and analyzing data precisely.
Introduction
Augmented designs are evaluating hundreds of germplasm lines in the same experiment, utilizing a minimal measure of experimental stuff, which are enough for one replicate only. Crop breeding programs use conventional approaches and biotechnological approaches provided huge quantities of experimental material to generate data to test. Large number of germplasms or new varieties called test treatments are analysis with comparison to widely cultivate superior varieties used as control treatments. Due to the limited quantity of experiment materials (test treatment) are not replicated in this design, but the optimum number of checks (control treatments) are replicated in each block so as maximize the efficiency per observations for test treatment vs. control treatment comparison.
The heterogeneity in soil fertility and disease, pest infestation oftenly found at experimental sites. So if the test treatment is grown in unreplicated manner, local variety required for analysis, adjust plot means for any environmental variability in experimental trial. Small block made to avoid the heterogeneity in the block and checks are replicated in a systematic manner. The replicated checks measure the variation in a trait across the trail and the observed the value of test treatment against its value of adjacent checks.
Augmented block design are two types, complete block and incomplete blocks for the check genotypes. Randomized complete block design, checks replicated equally in each block, whereas incomplete block design, block size are not same, i.e. an equal number of treatments not accommodated in each block.
Layout of augmented design
In experimental material huge quantity of data to the layout of randomization augmented design. Some symbols used to make easy to understand. u (number of control treatment), w (number of test treatment) and b (number of blocks in experiment).
For data are put to online to make a design layout by SAS or layout can be made manually. First the user enters the design parameter in, and replication of control treatment(s) to maximize the efficiency per observation is automatically computed.
In the hypothetical example, in that location are four check varieties (control treatment) denoted C1, C2, C3, C4 and 20 germplasm lines (test treatment) are denoted T1, T2, T3,………, T20 computed complete randomized block design.
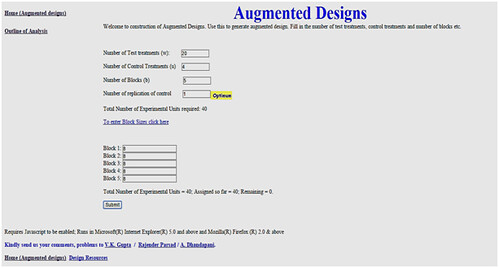
http: //www.iasri.res.in/design/Augmented%20Designs/home.htm
Layout of agumented design is given below:
Experimental Units
________________________________________
Block 1: (T6, T2, C4, C1, T12, C3, T8, C2)
________________________________________
Block 2: ( C3, C1, T4, T14, C2, T17, C4, T16)
________________________________________
Block 3: ( C2, C3, T10, C1, C4, T13, T20, T15)
________________________________________
Block 4: ( C2, C3, T9, C4, T7, C1, T5, T19)
________________________________________
Block 5: (T11, C1, C3, C4, T18, T3, T1, C2)
________________________________________
Analysis of data
Analysis is done by online software SPAD (statistical package for augment designs) software or web service. A partitioning of the degrees of freedom in an analysis of variance (ANOVA) table for this design is:
Source of variation - Degrees of freedom
Block - 4
Genotype - 23
Check - 3
New - 19
Check versus new - 1
B x check - 12
Correction for mean - 1
Total - 39
Web service gives you the opportunity to analyse your data without installing software to computer (Rathore, Parsad and Gupta, 2004).
The user enters data in specified format for analysis through augment block design. The online software available for analysis. For this treatment are renumerning as 1,2,….., u, u+1,……, u+w. Data should contain three columns first for block number, second for treatment and observed value in the third block. The data value separated by a TAB. If data already prepared in Excel sheet in columns than only paste the data online to get analysed.
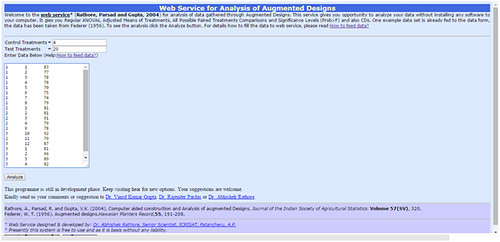
http://www.iasri.res.in/SpadWeb/
The analysis data gives ANOVA for Adjusted means of treatments and ANOVA for Adujusted means of block. It gives values of R-square, coefficient of variation, Root mean sum of square of error and General mean. It also gives all possible paired treatment comparisons and significance levels (Prob>F) and Critical difference between two controls, two test treatment (in a same block and different block separately) and in between test treatment and control treatment at 5% and 1% separately if it's possible to calculate.
SPAD is also offered two more type contrast analysis. The GBD test for test vs controls and user defined contrast analysis
Interpreted the values of analysis
In the analysis of variance treatment means with at least one word same are at par or not significantly different. In case treatment effect is at par than no pairwise analysis generated. There are not useful to compute multiple comparison test when treatment effects are at par.
From the table, highest value (>0.05) in paired treatment comparison shows significantly different from paired value and lower value (<0.05) in paired treatment comparison shows at par from paired values.
The treatment divided into Tests, Controls and Tests vs Controls, the tests and Tests vs Controls are significantly different If Prob > 0.05 instead of at par if Prob < 0.05. This exhibit that test treatments are performing differently and there is a significant difference between test and control treatments.
References:
1. Abhishek Rathore, Rajender Parsad and VK Gupta (2004). Computer aided construction and analysis of augmented designs. Journal of Indian Society of Agricultural Statistics, 57 (special volume), 320-344.
2. http://www.iasri.res.in website of Indian Agricultural Statistics Research Institute.
About Author / Additional Info:
Division of Plant breeding and Genetics
Sher-e-Kashmir University of Agricultural Sciences and Technology of Jammu (SKUAST-Jammu), India.